Navigating the Complex Landscape of AI and Information Bias
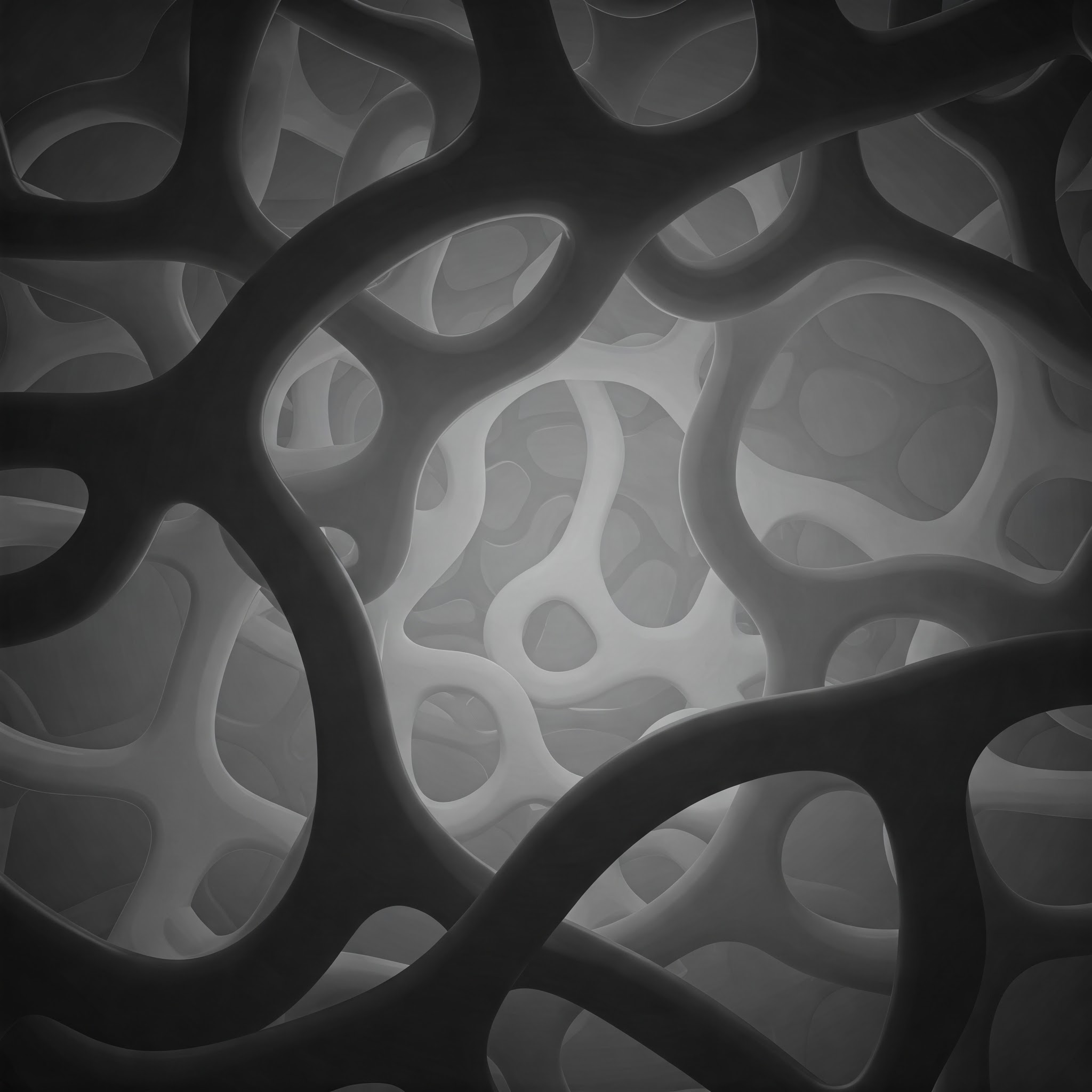
Title: Navigating the Complex Landscape of AI and Information Bias
Introduction
Artificial Intelligence (AI) has become an integral part of our daily lives, influencing everything from the news we read to the products we buy. However, as AI systems increasingly mediate our access to information, concerns about information bias have grown. Information bias in AI refers to the systematic errors introduced into data collection, processing, and interpretation, which can lead to skewed or unfair outcomes. This blog post explores the nature of AI-driven information bias, its implications, and strategies to mitigate its effects.
Understanding Information Bias in AI
Information bias in AI can manifest in various forms, often stemming from the data used to train these systems. AI models learn from historical data, and if this data contains biases, the AI will likely perpetuate or even amplify these biases. For example, if a job recruitment AI is trained on data from a company that historically favored male candidates, the AI might continue to favor male applicants, thereby reinforcing gender bias.
Types of Information Bias in AI
-
Selection Bias: This occurs when the data used to train an AI model is not representative of the population it is supposed to serve. For instance, facial recognition systems trained predominantly on lighter-skinned individuals may perform poorly on darker-skinned faces.
-
Confirmation Bias: AI systems can develop this bias when they are designed to confirm pre-existing beliefs or hypotheses. This is particularly problematic in recommendation systems that suggest content aligning with users' existing views, creating echo chambers.
-
Measurement Bias: This type of bias arises from errors in data collection methods. For example, if a health monitoring app primarily collects data from young, tech-savvy individuals, it may not accurately represent the health metrics of older populations.
-
Algorithmic Bias: This occurs when the algorithms themselves are designed in a way that introduces bias. For example, an algorithm that prioritizes cost-saving in healthcare might disproportionately affect low-income patients by denying them necessary treatments.
Implications of AI-Driven Information Bias
The consequences of information bias in AI are far-reaching and can affect various sectors:
- Social Justice: Biased AI systems can perpetuate social inequalities by reinforcing stereotypes and discriminatory practices.
- Economic Impact: Businesses relying on biased AI may make flawed decisions, leading to financial losses and reduced consumer trust.
- Public Safety: In critical areas like law enforcement and healthcare, biased AI can lead to wrongful arrests or misdiagnoses, endangering lives.
Strategies to Mitigate Information Bias in AI
Addressing information bias in AI requires a multi-faceted approach:
-
Diverse and Representative Data: Ensuring that training datasets are comprehensive and representative of all relevant demographics can help reduce selection bias.
-
Bias Audits: Regularly auditing AI systems for bias can help identify and rectify issues before they cause harm. This involves both technical assessments and ethical reviews.
-
Transparency and Explainability: Making AI algorithms more transparent and understandable can help stakeholders identify potential biases and understand how decisions are made.
-
Inclusive Design: Involving diverse teams in the development of AI systems can bring multiple perspectives to the table, reducing the likelihood of biased outcomes.
-
Regulatory Frameworks: Governments and organizations should establish guidelines and regulations to ensure that AI systems are developed and deployed responsibly.
Conclusion
AI has the potential to revolutionize how we access and interpret information, but it also poses significant challenges related to information bias. By understanding the types of bias that can occur and implementing strategies to mitigate them, we can harness the power of AI while minimizing its risks. As we continue to integrate AI into various aspects of society, it is crucial to remain vigilant and proactive in addressing these issues to ensure fair and equitable outcomes for all.
Call to Action
What are your thoughts on AI and information bias? Have you encountered instances where AI seemed biased? Share your experiences and join the conversation in the comments below. Let's work together to create a more equitable AI-driven world.
This blog post aims to provide a comprehensive overview of the issue, encouraging readers to think critically about the role of AI in shaping information and to take action towards more ethical AI practices.